- 글쓴시간
- 분류 기술,IT/스테이블 디퓨전
정복이란 "의식을 행할때 입는 예복"을 말한다. 여성용 정복이라함은 블레이저, 스커트, 블라우스, 스타킹, 펌프스 정도로 되어있지만 조금 다르게 생성해 보았다.
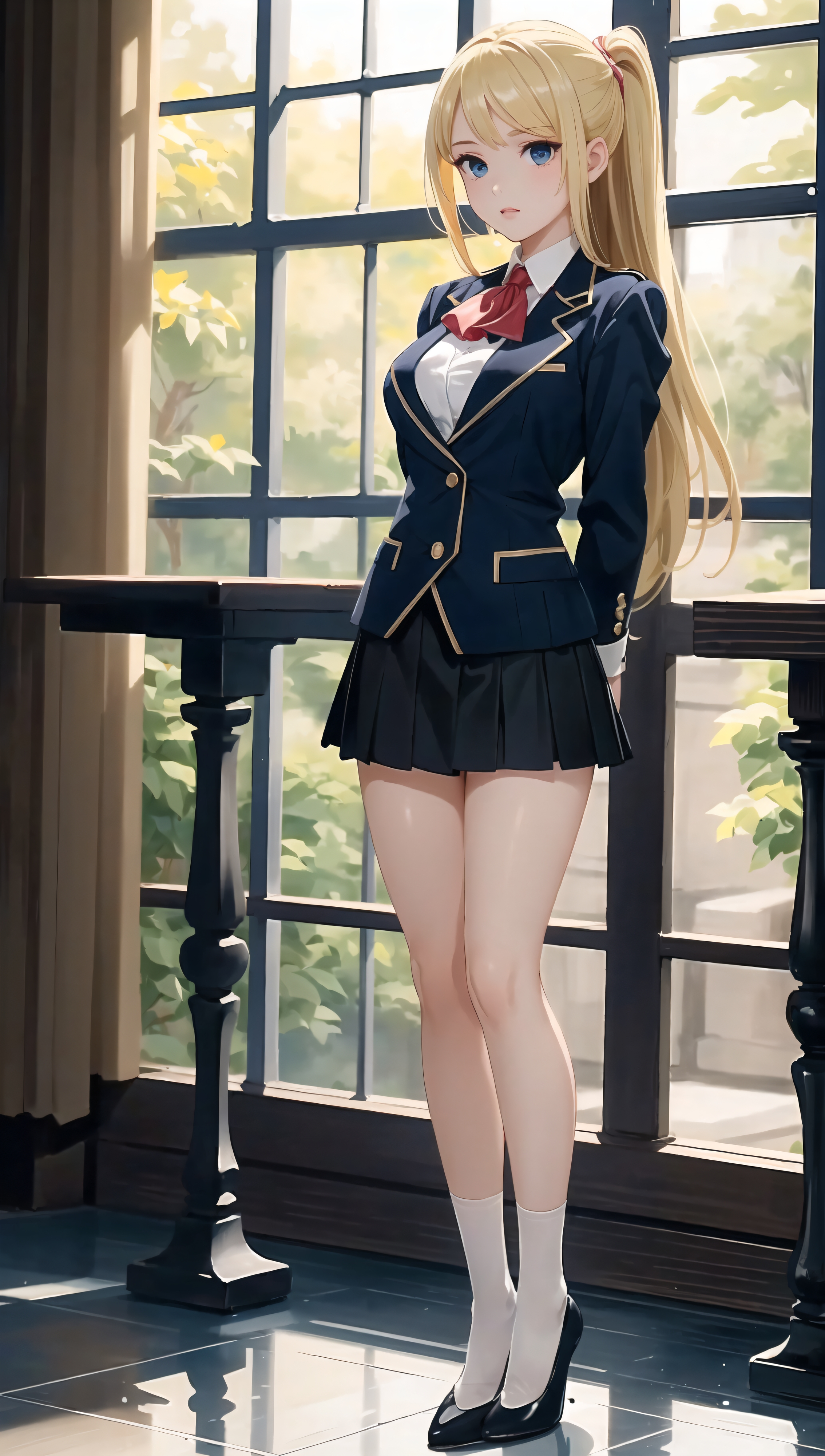
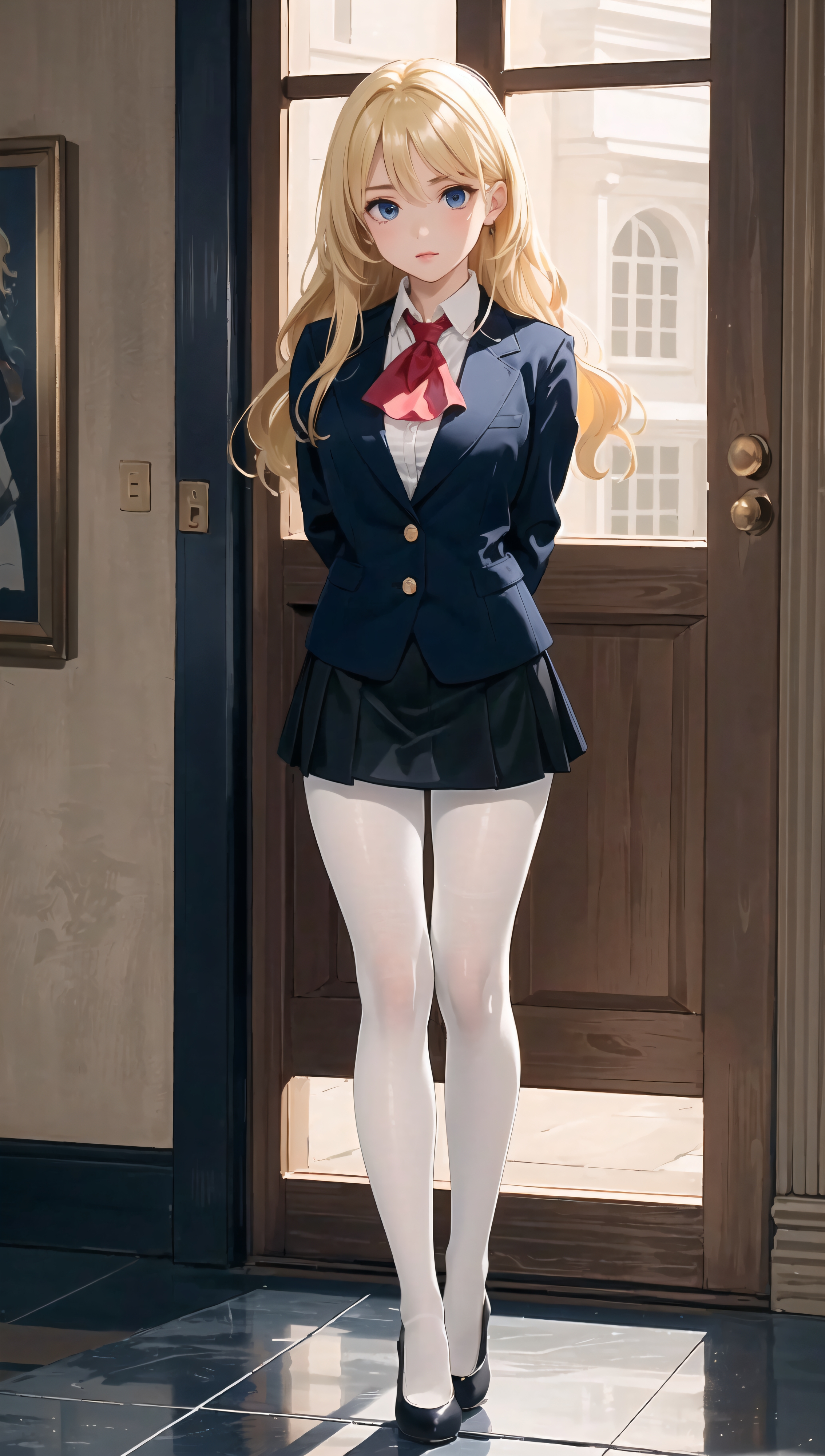
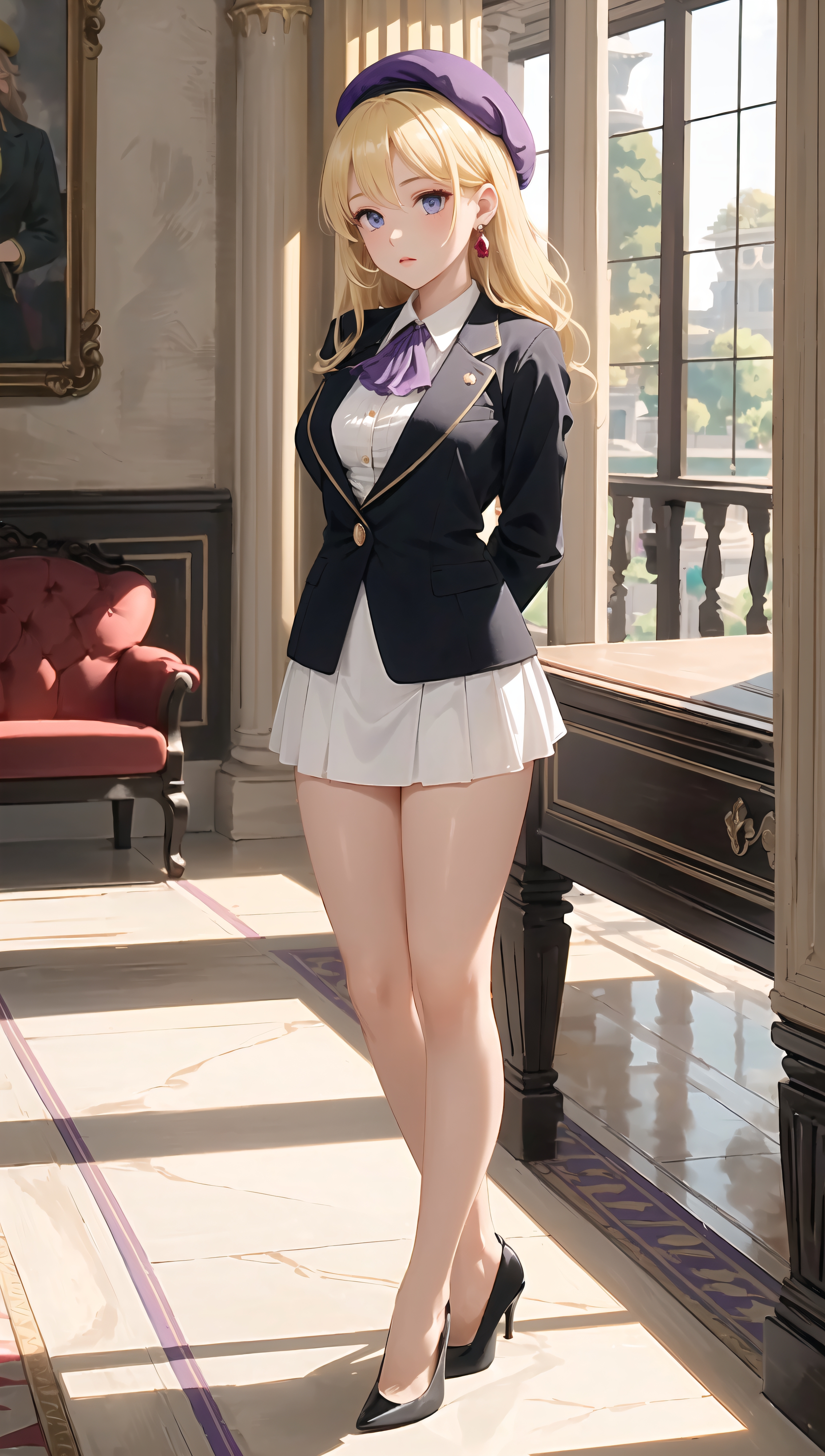
윈디하나의 누리사랑방. 이런 저런 얘기
100%|████████████████████████████████████████████████████████| 24/24 [02:33<00:00, 6.40s/it]
Total progress: 100%|██████████████████████████████████████████████| 24/24 [02:36<00:00, 6.54s/it]
Total progress: 100%|██████████████████████████████████████████████| 24/24 [02:36<00:00, 6.36s/it]
+-----------------------------------------------------------------------------------------+
| NVIDIA-SMI 550.67 Driver Version: 550.67 CUDA Version: 12.4 |
|-----------------------------------------+------------------------+----------------------+
| GPU Name Persistence-M | Bus-Id Disp.A | Volatile Uncorr. ECC |
| Fan Temp Perf Pwr:Usage/Cap | Memory-Usage | GPU-Util Compute M. |
| | | MIG M. |
|=========================================+========================+======================|
| 0 NVIDIA GeForce GT 1030 Off | 00000000:01:00.0 Off | N/A |
| N/A 53C P0 N/A / 19W | 1955MiB / 2048MiB | 100% Default |
| | | N/A |
+-----------------------------------------+------------------------+----------------------+
+-----------------------------------------------------------------------------------------+
| Processes: |
| GPU GI CI PID Type Process name GPU Memory |
| ID ID Usage |
|=========================================================================================|
| 0 N/A N/A 5895 C python3 1952MiB |
+-----------------------------------------------------------------------------------------+
제품 시리즈 버전 출시일최종갱신일: 2024.04.21
------------------------------ ------------- -------
RIVA TNT, TNT2, Geforce 256, 2 71.86 2005.xx
GeForce 2 MX,3,4 96.43 2012.09
GeForce 5 FX 169.12 2008.02
GeForce 6, 7 304.137 2017.09
GeForce 8, 9,100,200,300 340.108 2019.12
GeForce 400,500 390.157 2022.11
GeForce 600,700 470.239.06 2024.02
GeForce 900 ~ 550.76 2024.04